 |
Theory/Methodology

Click to see QuickTime movie
Merging Phylogeography and Paleoecology
Current work in phylogeography involves developing rigorous
statistical methods that have the power to test alternative
population histories characterized by complex and heavily
parameterized models. To this end, the Moritz group is focusing
on two approaches: 1) Markov Chain Monte Carlo (MCMC); and
2) approximate Bayesian inference (AB; Estoup et al. 2004).
Both approaches are done with the impetus of integrating spatial
genetic data with other historical and ecological information
into one analysis in order to improve our ability to infer
biogeographic histories across taxa within a community. Additionally,
such an analysis can test if present ecological and physiological
characteristics of a species can correctly predict how it
reacts to climate change. A Bayesian framework is a natural
way to use such non-genetic information as prior distributions
for parameters of interest, while the genetic data can be
used to estimate parameters by via posterior distributions,
such that alternate histories can be compared by comparing
the posterior estimates under alternative prior distributions.
Currently, MCMC approaches are enabling us to test spatially
explicit phylogeographic models in 2D habitats. Alternatively,
the AB approach allows a flexible framework to investigate
a wide range of biogeographic hypotheses, while sacrificing
some of information used in an MCMC analysis.
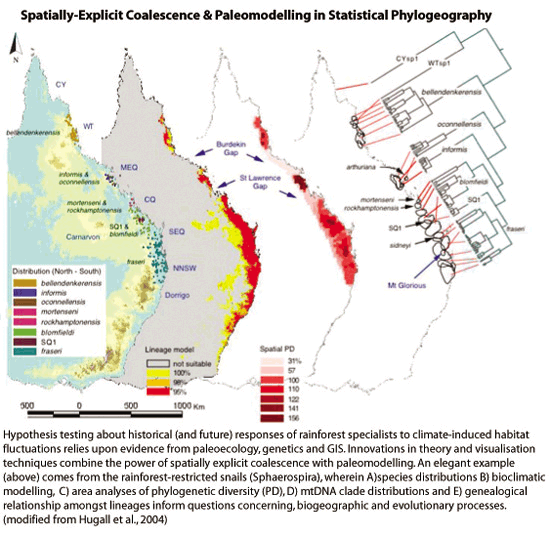
Dancing Trees (Stuart Baird)
Motivated by an interest in broader scale evolutionary inference,
and in consultation with Ian Wilson, a complementary set of
algorithms has recently been developed which allows generalization
over a wider class of models of population structure. The
approach achieves this generality with a trade-off against
computation time. A Markov chain Monte Carlo simulation is
created with a state consisting of a tree of paths through
discrete space and time. Movement is on a two-dimensional
stepping-stone lattice. Between discrete opportunities for
movement demes are undisturbed by migration events, and so
coalescent probabilities can be described following standard
coalescent theory. Proposed transition on the chain state
can most succinctly be described as a series of dance steps
allowing nodes and paths on the tree to be moved in space
and time. The transitions are designed such that change in
the tree state is localized, bounded by the nodes connected
to the part of the tree being moved and consistent with the
stepping-stone paradigm. The process of the Markov chain Monte
Carlo simulation can be visualized by iterating the chain
and sampling the positions of the lineage paths that make
up the tree. Animating the resulting snapshots of the state
suggests a label for this approach: the dancing trees algorithm.
The dancing trees algorithm can be used to define better the
set of lineage trees in nature whose history is well approximated
by the population splitting model. In summary the current
work has wide implications: the authors' approach and its
complements pave the way towards a sounder understanding of
population structure and the evolutionary process.
Phylogeographic Experimental Design
Using phylogeographic data to test different biogeographic
histories has generally relied on collecting the easily obtained
mtDNA in multiple co-distributed taxa. However, the resulting
parameter estimates implicit in such biogeographic hypotheses
suffer from substantial stochastic error because they are
based on a single linked genealogical history per taxon. For
instance, mtDNA-based divergence time estimates often show
wide variation across co-distributed taxa-pairs that are likely
to have diverged nearly simultaneously. Despite the commonly
stated limitations of single mtDNA datasets, there has been
limited statistical guidance into how researchers should design
phylogeographic studies. Therefore we are involved in determining
optimal sampling strategies with respect to the number of
loci, spatial coverage and number of individuals by conducting
Power analyses for different data types (mtDNA, introns, SNPs)
using simulations .
|
 |